AI Presents Thorny Regulatory Questions
UA law professor Jane Bambauer thinks a measured approach will be best in taming the ‘Wild West’ of artificial intelligence
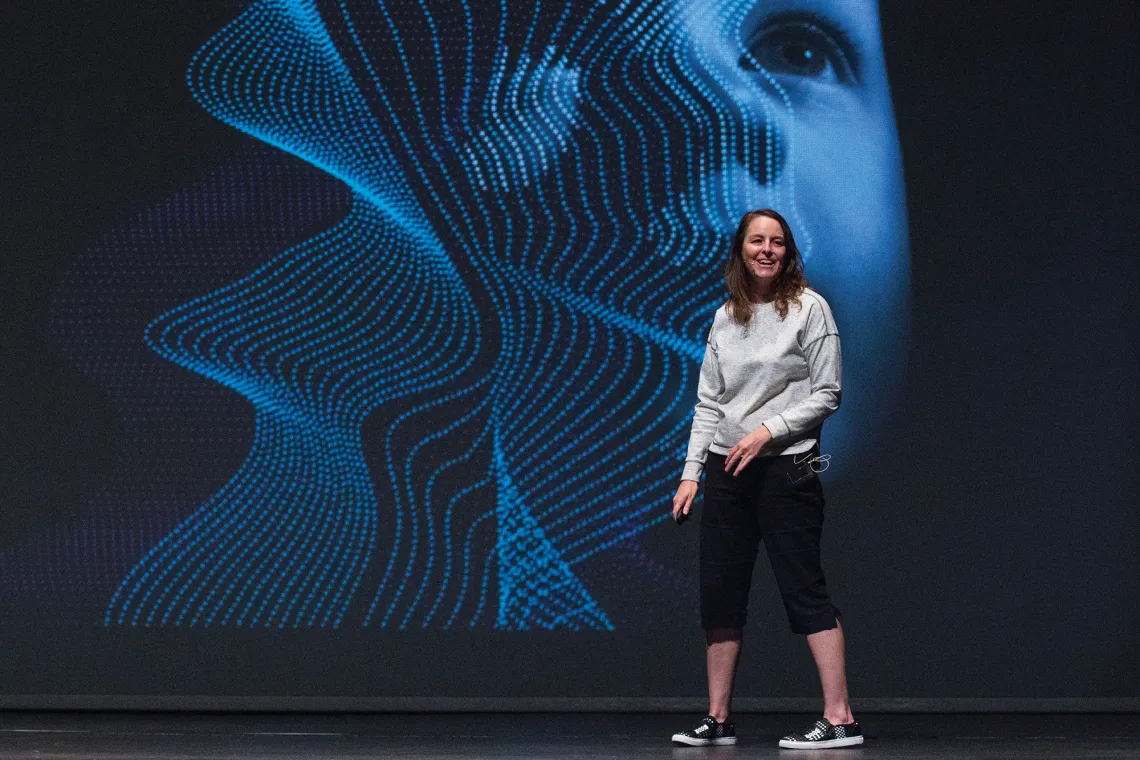
Jane Bambauer: “My impulse is to let companies figure out what’s working and what isn’t, before we regulate.”
If it continues in its lane, it will plow into several pedestrians. If it swerves into the adjacent lane, it will ram into a concrete barrier. In either case, injuries and even loss of life are likely.
Although this scenario currently lives only on Moral Machine, a platform designed by the Massachusetts Institute of Technology to “gather a human perspective on moral decisions made by machine intelligence,” it might be coming soon to a street near you. And that has
Jane Bambauer both fascinated and concerned by what she describes as the current “Wild West” of artificial intelligence.
“The coordination of a world with both driven cars and driverless cars will be incredibly complicated,” Bambauer, a University of Arizona law professor, told a group of science teachers and graduate students after her lecture in February on “Machine Influencers and Decision Makers” at Centennial Hall. The lecture was the fifth in the College of Science series on “Humans, Data and Machines,” which has focused on the convergence of the digital, physical and biological worlds.
Bambauer said the transportation industry will be turned upside down in the same way that it was when automobiles disrupted a world of horse-drawn carriages, forcing both modes to share the road. She said it will be tempting to rush in and regulate — but much better to go slowly.
“My default position with new technology is not to do much heavy-handed regulation,” she told the teachers and students.
“I’m a bit of a contrarian in my field. My impulse is to let companies figure out what’s working and what isn’t, before we regulate. There are instances where, when trying to regulate in advance, you end up missing on innovations (that follow),” she said, citing the early World Wide Web as an example.
Bambauer told her audience that it’s useless trying to fight the onslaught of algorithms. They’re pervasive, they’re not going away, and they’re assessing our credit scores, career interests, health care and more.
“We interact with machine-learning algorithms almost any time we do anything on the internet,” she said.
It’s more prudent, then, to ask probing questions such as: Is an algorithm biased? Is it manipulative? Are there hidden moral or political issues?
An example of bias, Bambauer said, is seen in how scores from the computer program COMPAS (Correctional Offender Management Profiling for Alternative Sanctions) are used to create a risk scale for criminal recidivism. Although the program’s 137 variables do not include race or ZIP code, the scale still has bias built into its computations, as Bambauer demonstrated through a series of bar graphs.
Even the perception of bias can turn people away from trust in our institutions, she said, showing how basic Google searches can be interpreted by some as an indication of the tech giant’s political leanings.
Manipulation can surface in something as innocuous as food reviews or as insidious as “fake news.” Facebook’s news-feed algorithm “has incredible power,” Bambauer said, adding that “the filter bubble is limiting you” in terms of big-picture perspective.
In any event, algorithms are complicated to untangle.
“All of the problems (with algorithms) are interconnected,” she said. “Accuracy might increase bias. Data gathering might affect privacy. The problems are with setting priorities among competing goals.”
Echoing what previous speakers in the series had said, she noted, “It’s easy to blame the algorithms, but algorithms do what we ask.”